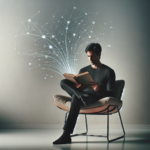
How I Read Books in the Era of Artificial Intelligence
January 18, 2025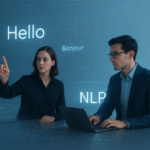
Uncovering Natural Language Processing (NLP): Challenges and Opportunities in AI Competitions
April 26, 2025Introduction
Generative AI in content creation and beyond is reshaping industries at a rapid pace. From design and advertising to healthcare and education, AI can now produce text, images, music, and even video content with minimal human input. This article explores how generative AI works, where it’s being applied, and what it means for the future of innovation, ethics, and society.
What Is Generative AI?
Generative AI refers to a subset of artificial intelligence that focuses on creating new content—whether it’s text, images, audio, or code. Unlike traditional machine learning models that predict or classify, generative AI actively produces original outputs based on learned data patterns.
This paradigm shift from passive analysis to active generation is what makes generative AI so powerful—and disruptive.
Key Generative AI Models and Techniques
-
Generative Adversarial Networks (GANs)
GANs use a generator and discriminator to produce realistic outputs by learning to fool the system. Commonly used for image generation and deepfakes. -
Variational Autoencoders (VAEs)
VAEs compress and reconstruct data, allowing for new variations that maintain the characteristics of the original data set. -
Transformer-Based Models (like GPT)
Transformers use self-attention to generate human-like text and can be applied to text-to-image, code generation, and even music composition.
Generative AI in Content Creation
1. Text Generation and Copywriting
-
Blog writing, ad copy, email campaigns, and news summaries
-
Natural-sounding language translation
2. Image and Design Generation
-
AI art and illustrations via tools like DALL·E or Midjourney
-
Rapid prototyping and design mockups
3. Audio and Music
-
AI-generated music for games and podcasts
-
Realistic voice synthesis for audiobooks and virtual assistants
4. Video and Animation
-
Deepfakes and avatar creation
-
Automated animation of characters or objects
Going Beyond Content Creation
1. Healthcare and Drug Discovery
-
Designing molecules and accelerating the drug discovery pipeline
-
Enhancing medical imaging datasets while preserving privacy
2. Robotics and Manufacturing
-
Product shape optimization and virtual environment training
-
Cost reduction in prototyping and deployment
3. Urban Planning and Architecture
-
Smart blueprint generation
-
Simulating traffic and energy use in real-time environments
4. Education and Training
-
Personalized, adaptive learning content
-
AI-powered tutoring and assessments
Ethical and Societal Implications
1. Bias and Fairness
AI models may reflect and reinforce harmful biases found in training data.
2. Misinformation and Deepfakes
Realistic fake media can undermine trust—raising the need for detection and governance.
3. Data Privacy
Scraping massive datasets may compromise personal data if not properly governed.
4. Intellectual Property
AI-generated work often includes fragments of copyrighted materials—posing legal questions about ownership.
5. Environmental Concerns
Training large-scale models requires high energy consumption and raises sustainability issues.
Conclusion: The Road Ahead for Generative AI
The future of generative AI in content creation and beyond is both thrilling and challenging. It promises faster innovation, greater personalization, and wide-reaching productivity—but it also demands ethical responsibility.
As these technologies become more embedded in our lives, it’s crucial that researchers, developers, and policymakers work together to ensure their impact is fair, transparent, and sustainable.
Generative AI is not just transforming how we create—it’s redefining how we think, design, and live.
References
- Brown, T., Mann, B., Ryder, N., Subbiah, M., Kaplan, J., Dhariwal, P., … & Amodei, D. (2020). Language Models are Few-Shot Learners. arXiv preprint arXiv:2005.14165.
- Gómez-Bombarelli, R., Wei, J. N., Duvenaud, D., Hernández-Lobato, J. M., Sánchez-Lengeling, B., Sheberla, D., … & Aspuru-Guzik, A. (2018). Automatic chemical design using a data-driven continuous representation of molecules. ACS Central Science, 4(2), 268-276.
- Goodfellow, I., Pouget-Abadie, J., Mirza, M., Xu, B., Warde-Farley, D., Ozair, S., … & Bengio, Y. (2014). Generative Adversarial Networks. arXiv preprint arXiv:1406.2661.
- Kingma, D. P., & Welling, M. (2013). Auto-Encoding Variational Bayes. arXiv preprint arXiv:1312.6114.
- Vaswani, A., Shazeer, N., Parmar, N., Uszkoreit, J., Jones, L., Gomez, A. N., … & Polosukhin, I. (2017). Attention Is All You Need. arXiv preprint arXiv:1706.03762.